How to prevent buildings collapse by training the AI’s “neural network”
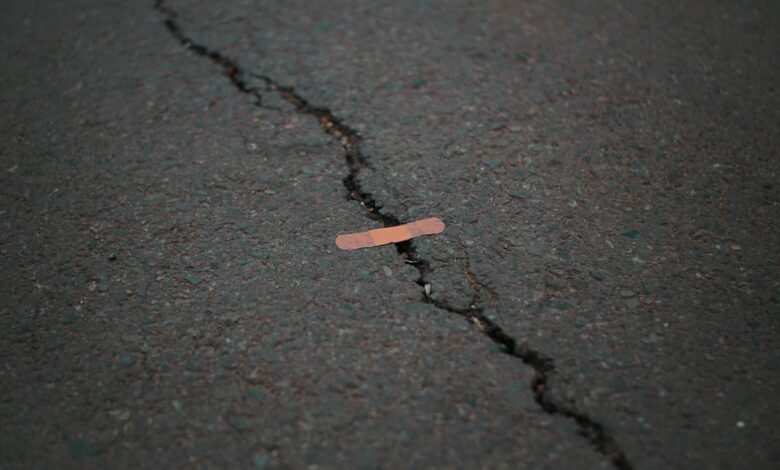
The experiments developed by the team have made it possible to identify believes smaller than a hundredth of a millimeter
(sustainabilityenvironment.com) – Our built environment is aging. Every day, houses, bridges and roads increase more and more the possibility that they are affected by structural failure or cracks dangerous for the safety of those who live in these places. But how to prevent buildings collapse or the collapse of infrastructure if the number of building elements that would need a diagnosis grows daily? The solution could once again come from a clever use of artificial intelligence and robotics.
Train a robotic assistant to recognize problems
Starting from this great question, Drexel University College of Engineering researchers are trying to provide a robotic assistant with the information needed to support human inspectors in monitoring to prevent buildings collapse and infrastructure. The new ingredient added by the team uses machine learning to identify and inspect problem areas in a significantly shorter time than a human inspector could. The results of their research are published in the journal Automation in Construction.
Drexel University’s multi-scale system combines machine vision with a deep learning algorithm to identify problem areas of cracking. Subsequently, laser measurements of the affected regions are carried out, generating a “digital twin” of what has been scanned. In this way, it will be possible to identify and assess the more or less serious damage that could affect the system.
Increase inspection speed to prevent buildings collapse and bridges
“Cracks can be regarded as a patient’s medical symptoms that should be screened in the early stages,”, wrote the authors, Arvin Ebrahimkhanlou, Ph.D., assistant professor, and Ali Ghadimzadeh Alamdari, assistant researcher, both at Drexel’s College of Engineering. “Consequently, early and accurate detection and measurement of cracks are essential for timely diagnosis, maintenance, and repair efforts, preventing further deterioration and mitigating potential hazards“.
The problem, however, is that the number of structures that should be examined increases daily, transforming prevention into a sort of “health emergency” of structures in construction, but for which there are fewer and fewer skilled workers capable of dealing with it.
“Civil infrastructures include large-scale structures and bridges, but their defects are often small in scale,” Ebrahimkhanlou said. “We believe taking a multi-scale robotic approach will enable efficient pre-screening of problem areas via computer vision and precise robotic scanning of defects using nondestructive, laser-based scans“.
How the system works
Instead of a physical measurement interpreted “subjectively by the human eye“, the system uses a high-resolution camera with stereoscopic depth of the structure, connected to a deep learning program called “Neural Network“. Although the scientific explanation may seem complex, we are dealing with these kinds of programs daily: these are systems used for facial recognition, deepfake detection or drug development, systems capable of detecting the most subtle anomalies and discrepancies by analyzing huge volumes of data. Once the AI algorithm is “trained” to recognize data sets, it will be able to identify cracks or cracks to prevent buildings collapse.
The neural network is trained on a sample crack data set and is able to identify crack-like patterns in the images that the robotic system collects from the surface of a concrete structure,” explains Ebrahimkhanlou.
read also Sustainable concrete thanks to lead melting waste: the results of the Australian study
Once the region affected by the cracking has been identified, the system directs the robotic arm to scan it creating a 3D image of the damaged area. At the same time, a lidar camera (Light Detection and Ranging) scans the structure surrounding the crack. Combining both graphs creates a digital model of the area that shows the width and size of the crack and allows you to monitor changes between inspections over time.
“Tracking crack growth is one of the advantages of producing a digital twin model”. “In addition, it allows bridge owners to have a better understanding of the condition of their bridge, and plan maintenance and repair“, said Alamdari. In this way you have a better understanding of the conditions and you can better organize the maintenance and repair.
The experiments developed by the team of researchers have made it possible to identify believes smaller than a hundredth of a millimeter, surpassing in terms of performance cameras, scanners and high-quality devices. fiber optic sensors with a respectable margin. It is good to reiterate that, the final decision on intervention, will always have the human inspector, but that will be greatly facilitated by the work of the machine.